Ensuring Quality in AI: The Crucial Role of QA Testing
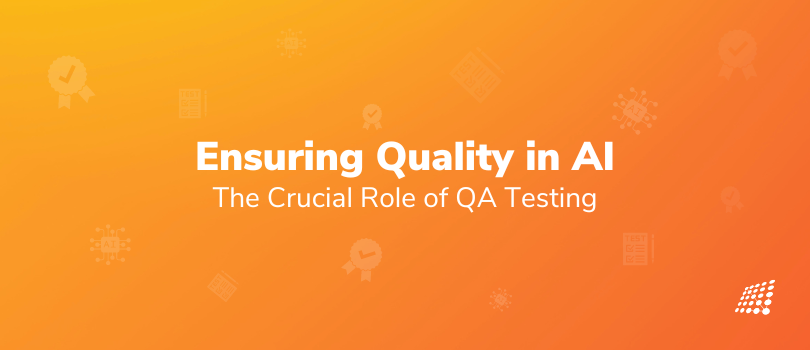
Industries are evolving that too quickly and fast thanks to Artificial Intelligence (AI), which is also radically altering how people use technology. It's become an irreplaceable component in many software programs due to its capacity to automate challenging tasks and improve human decision-making.
However, ensuring the quality of AI systems is not an easy task. To make sure that the AI system achieves the necessary performance standards, testing, and quality assurance must be done methodically. That's why, I thought it best to go into detail about the crucial role QA testing plays in ensuring the reliability of AI systems. So let's get started!
The Growing Importance of AI Testing for Software Applications
AI testing is a highly specialized field that calls for a particular set of skills and knowledge. That's what my experience as a QA professional has revealed to me. AI testing differs from standard software testing in that it assesses an AI system's capacity to learn from data inputs, adapt, and make predictions. This means that in addition to testing the software, AI testing also involves assessing the efficacy and performance of the AI models.
Understanding the Unique Challenges of AI Testing
Pay Attention to the Requirements Gathering Phase
QA testing should be a crucial component of the entire development lifecycle, beginning with the requirements-gathering stage. It helps validate the quality of an AI system. To thoroughly understand the functionality, performance requirements, and anticipated results of the AI system, the QA team should collaborate closely with the development team. This ensures that the AI system satisfies the desired performance standards and assists with spotting potential problem areas early in the development cycle.
Focus on Data Quality
Data quality is a crucial component of QA testing for AI systems. Data acts as the fuel that energizes AI systems. After all, the quality of data directly affects the accuracy and effectiveness of AI models. As a QA specialist, I have observed how bad data can produce biased results and inaccurate predictions. QA teams should carry out thorough data validation and verification tests, including data profiling, data cleansing, and data normalization, to assure data quality.
Emphasize Accuracy and Performance
QA testing for AI systems includes verifying the performance and accuracy of the AI models in addition to the data quality. This involves putting the models to the test with diverse data inputs and gauging how well they can pick up on and adjust to new information. As an example, let's utilize a chatbot that employs natural language processing (NLP) to communicate with users. The QA team should test the chatbot against diverse user inputs and assess its capacity to comprehend and react to various types of queries in order to assure the accuracy of the chatbot.
Evaluating AI Model for Bias
Testing for bias is vital when using QA testing for AI systems. AI models are developed using past data, which may be distorted inherently and result in unfair results. As a QA expert, I have observed how biased AI models can provide unjust outcomes and reinforce preexisting assumptions. QA teams should conduct thorough testing for fairness and examine the output of the AI model for any discriminating outcomes to reduce bias.
Security and Privacy
Furthermore, testing for security and privacy is part of QA for AI systems. AI systems' performance might be crucial to business operations because they may include sensitive data. Therefore, QA teams should conduct in-depth security testing to uncover potential weaknesses and guarantee that the data in the AI system is secure. Testing is done for things like data encryption, access control, and vulnerability assessments.
So you see, The quality of AI systems is ensured in large part by QA testing. The demand for thorough testing and quality assurance will only grow as AI continues to transform industries and permeate more software applications. To make sure AI systems satisfy the specified performance criteria, QA personnel must have a specialized skill set and knowledge in AI testing. We can make sure AI systems are accurate, unbiased, secure, and function as intended by using a methodical approach to testing and quality assurance.
Do you want to ensure the quality of your AI system? Look no further! Our team of experienced QA testers can help you achieve your desired performance criteria and offer expert quality assurance testing for your AI system efficiently.
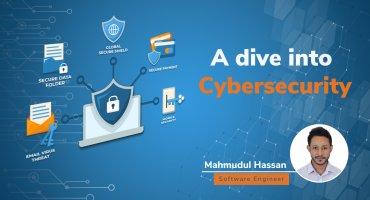
A Dive into Cybersecurity
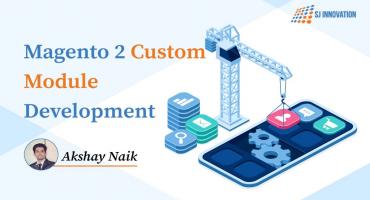
Magento 2 Custom Module Development
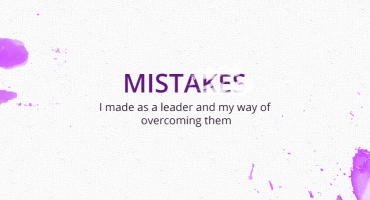