What You Need to Know about Integrating AI in SQA Processes
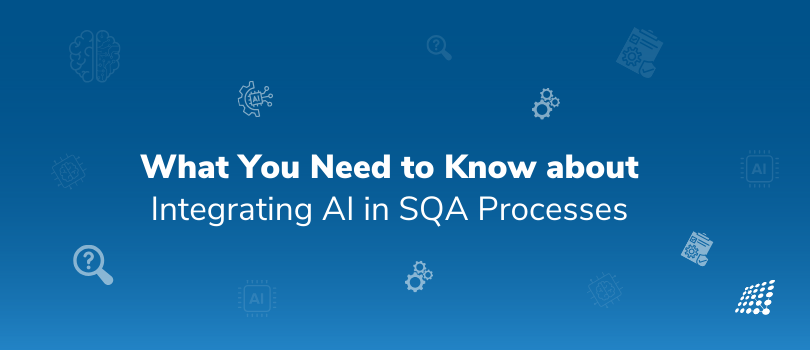
Quality Assurance always needs to meet the highest standards! While traditional Software Quality Assurance (SQA) processes have always been reliable, keeping pace with the complexities of modern software was a challenge. AI came as a savior, reshaping the very foundations of SQA. Here’s everything you need to know about integrating AI into SQA processes!
The Impact AI has on SQA
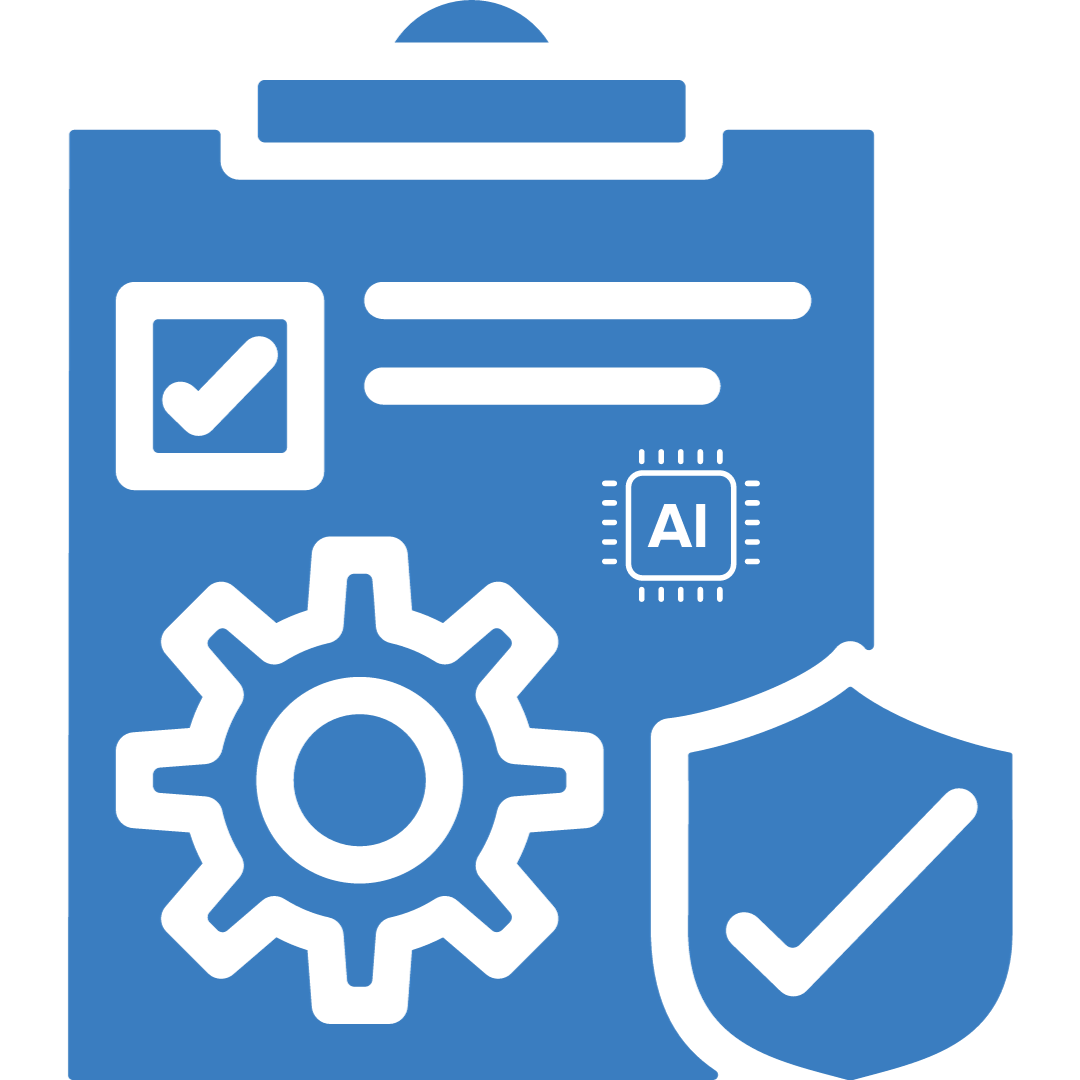
What is the impact AI has on SQA? To know this, you have to delve into the intricate interplay between these two fields. Traditional SQA, with its rigorous testing processes, has long been the guardian of software quality. However, as software applications grow in sophistication and intricacy, the need for innovative approaches becomes evident.
AI, with its machine learning capabilities, emerges as a potent ally in this quest for improved quality assurance. The fusion of AI and SQA introduces a paradigm shift, offering solutions to longstanding challenges in the software testing realm.
Integrating AI in SQA Processes
AI's Role in Test Case Generation
- A pivotal area where AI demonstrates its prowess is in the automation of test case generation.
- The traditional manual effort invested in crafting test cases is significantly reduced as machine learning algorithms take the helm.
- This shift towards intelligent test case creation not only bolsters test coverage but also introduces adaptability to changes within the software ecosystem.
- The result is a more efficient and dynamic testing phase that aligns seamlessly with the rapid evolution of software.
AI-driven Automation Frameworks
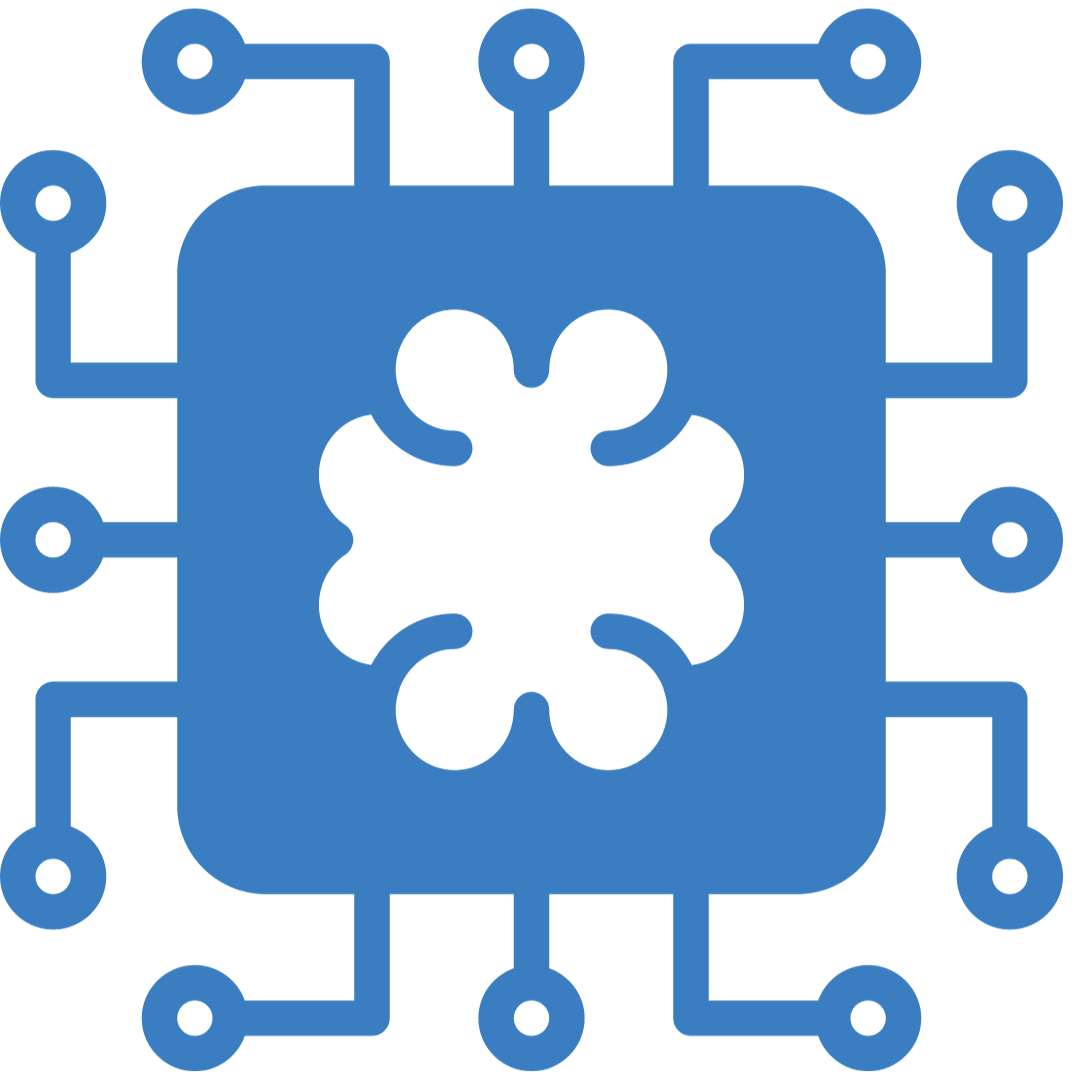
- The integration of AI goes beyond automation and extends to the very frameworks that underpin SQA.
- AI-driven test automation frameworks usher in a new era of adaptability and self-healing capabilities.
- These frameworks, infused with the intelligence of AI, not only keep pace with the dynamic nature of software development but actively contribute to the overall robustness of the testing process.
- The result is a more resilient and efficient testing framework that aligns with the demands of modern software development.
Predictive Analytics in Defect Prevention
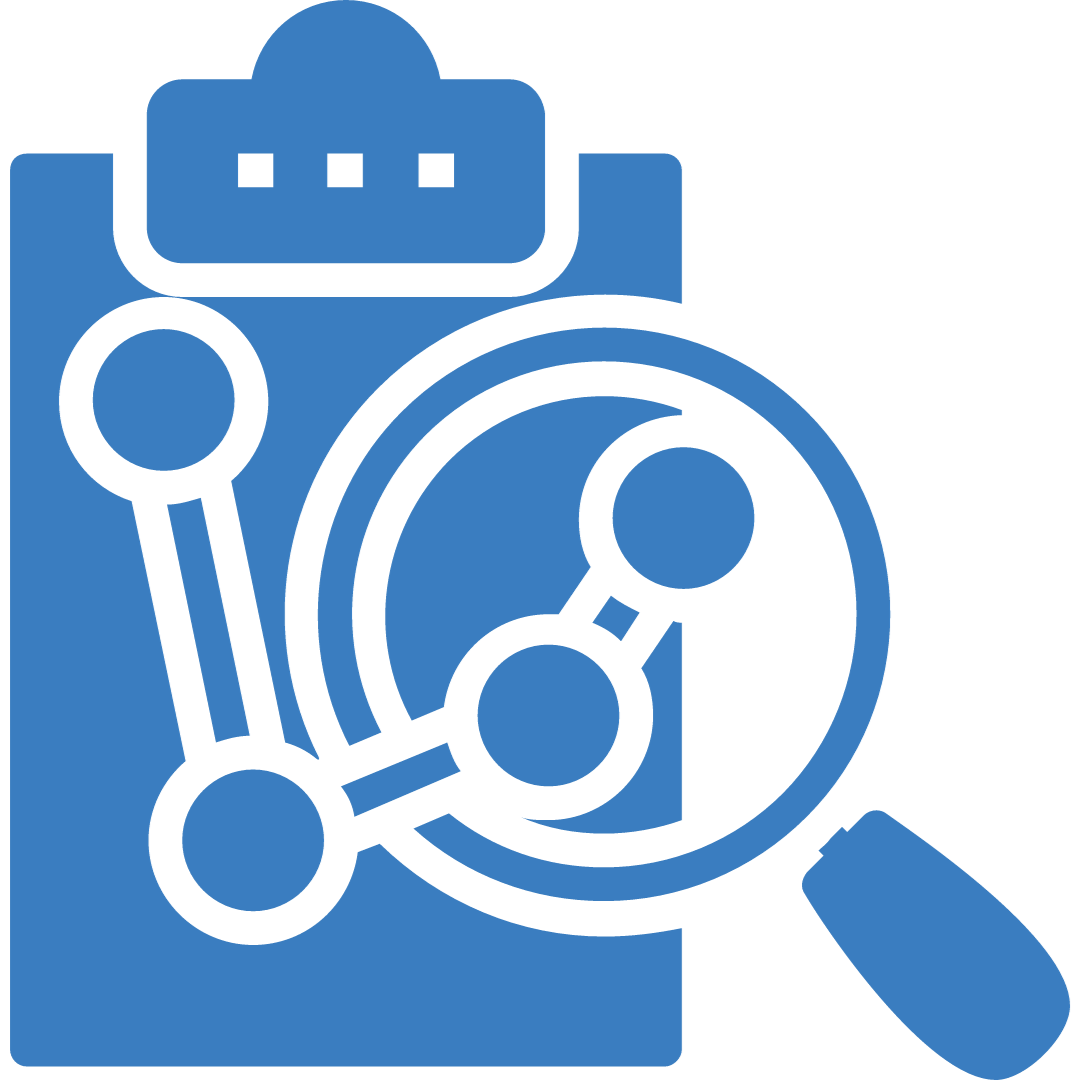
- Predictive analytics, empowered by AI, introduces a proactive dimension to defect prevention in SQA.
- By analyzing historical data and discerning patterns, AI models can identify potential defects before they manifest, allowing for preemptive measures.
- This shift from a reactive to a proactive approach in defect management marks a significant advancement, reducing the likelihood of defects impacting the end-user experience.
AI, Agile, and Continuous Testing
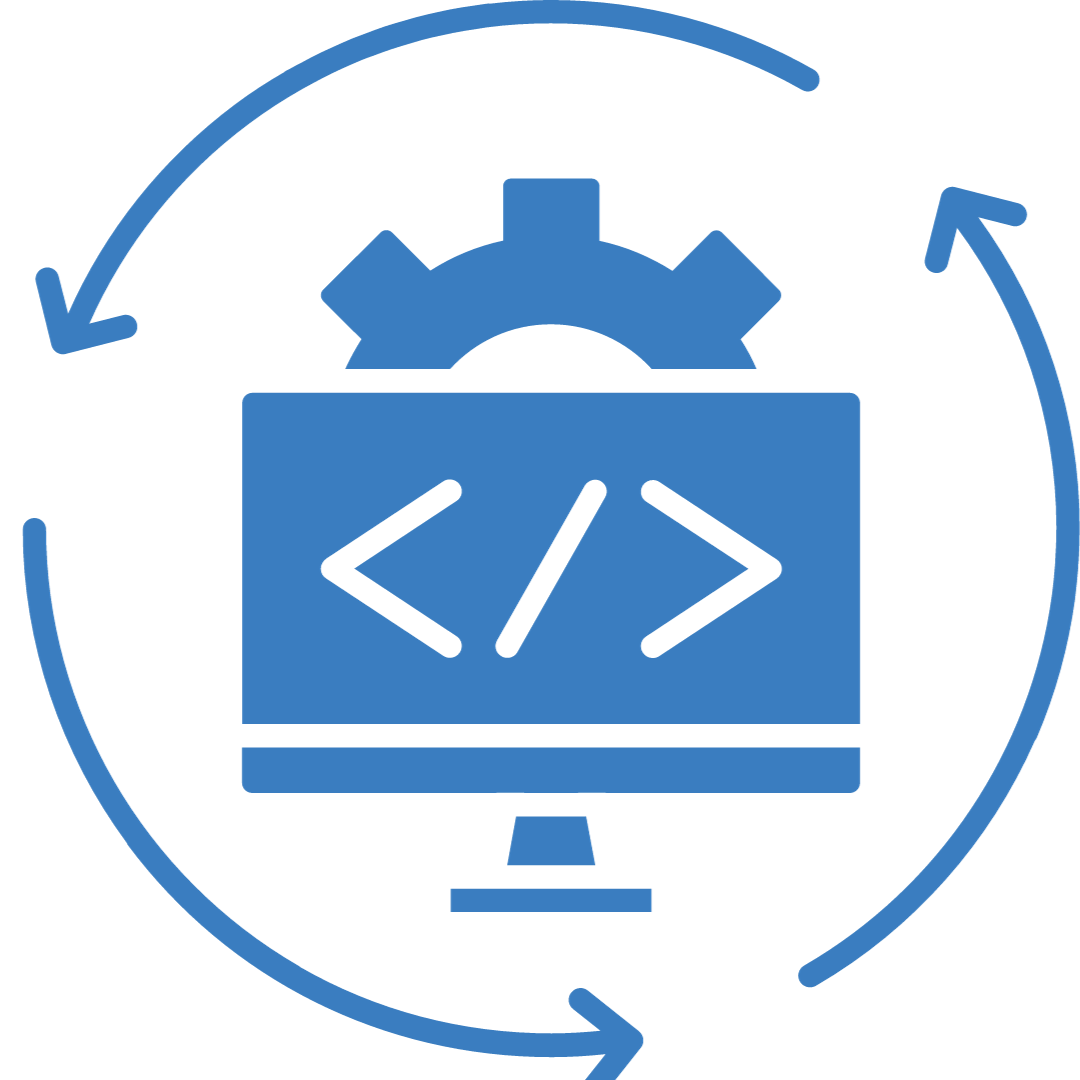
In the agile landscape, where rapid development cycles are the norm, the integration of AI seamlessly complements Agile principles. The synergy between AI and Agile enhances the speed and accuracy of testing processes, facilitating continuous testing in dynamic development environments. This blend of AI and Agile methodologies ensures that software quality is not compromised, even in the face of rapid iterations and changes.
AI's Contribution to Security
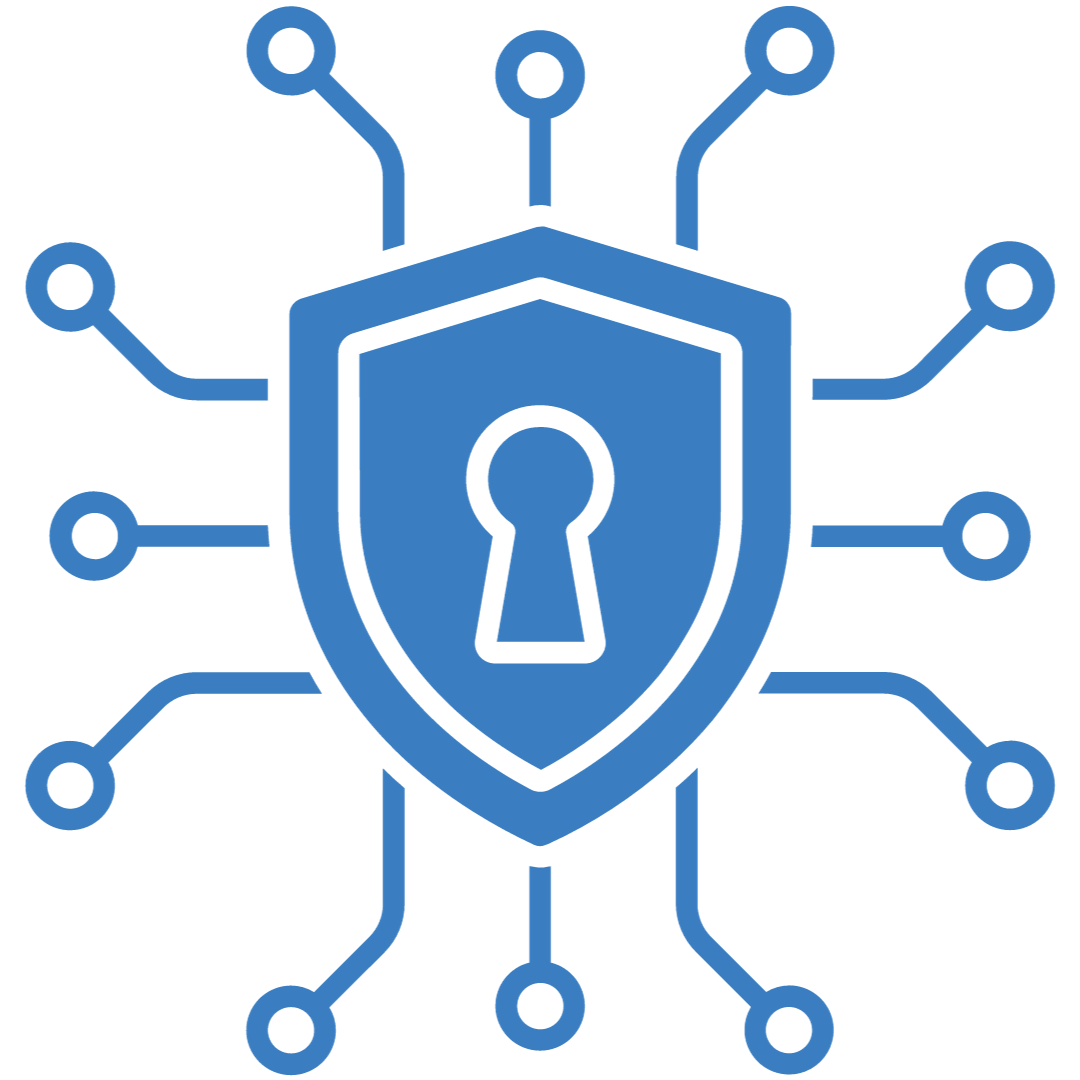
Security, a cornerstone of software quality, gains prominence with the integration of AI in SQA. AI plays a pivotal role in identifying and mitigating security vulnerabilities through advanced testing processes. As applications become more interconnected and prone to cyber threats, the incorporation of AI in security testing becomes indispensable. It is imperative, however, to maintain ethical considerations in this context, ensuring the responsible use of AI in safeguarding software applications.
Challenges and Ethical Considerations
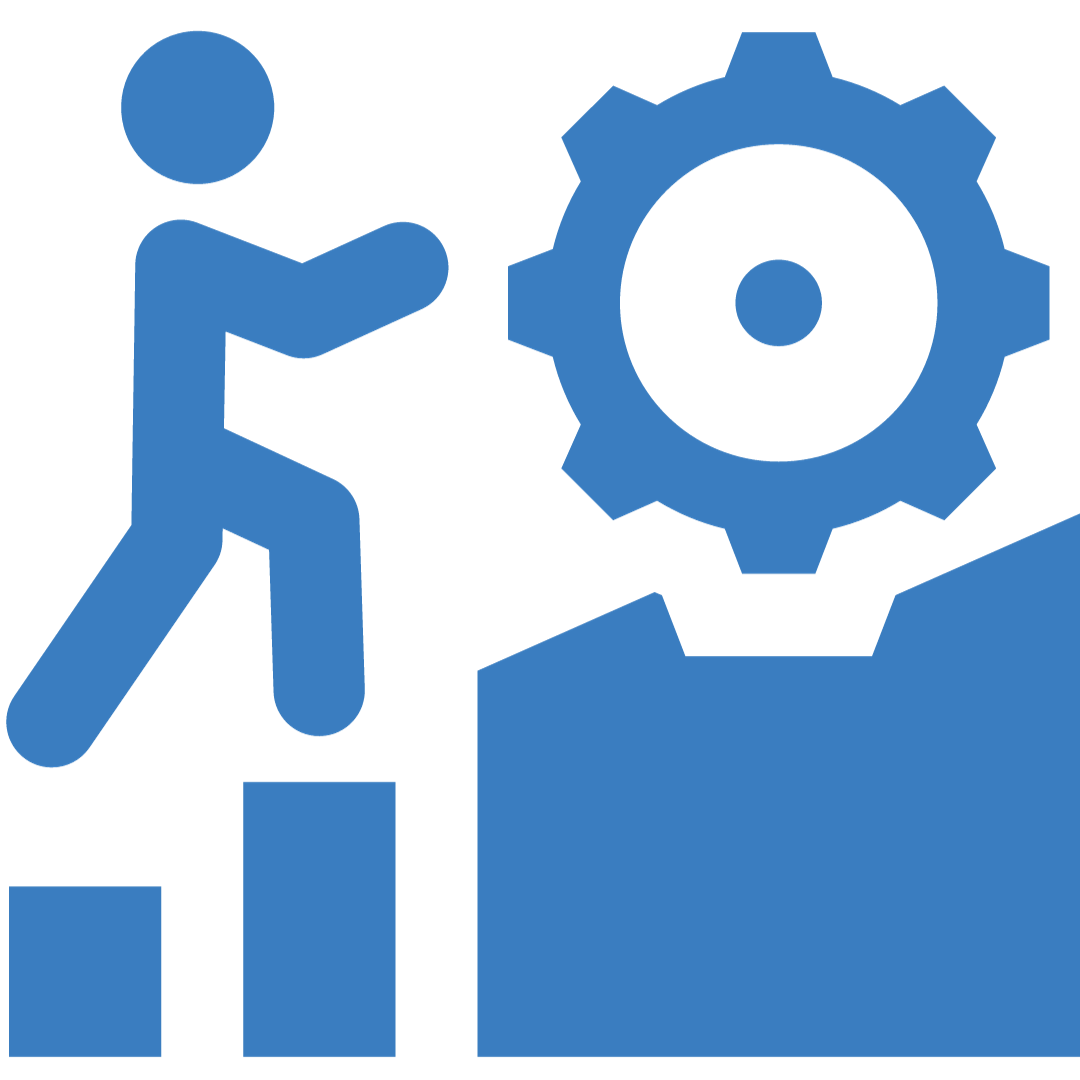
While the benefits of AI in SQA are evident, challenges persist. Issues such as data quality, model interpretability, and the potential for bias in testing require careful consideration. Addressing these challenges is imperative for the successful integration of AI into SQA processes, ensuring that the advantages of AI are harnessed responsibly and ethically.
The Future of SQA and AI
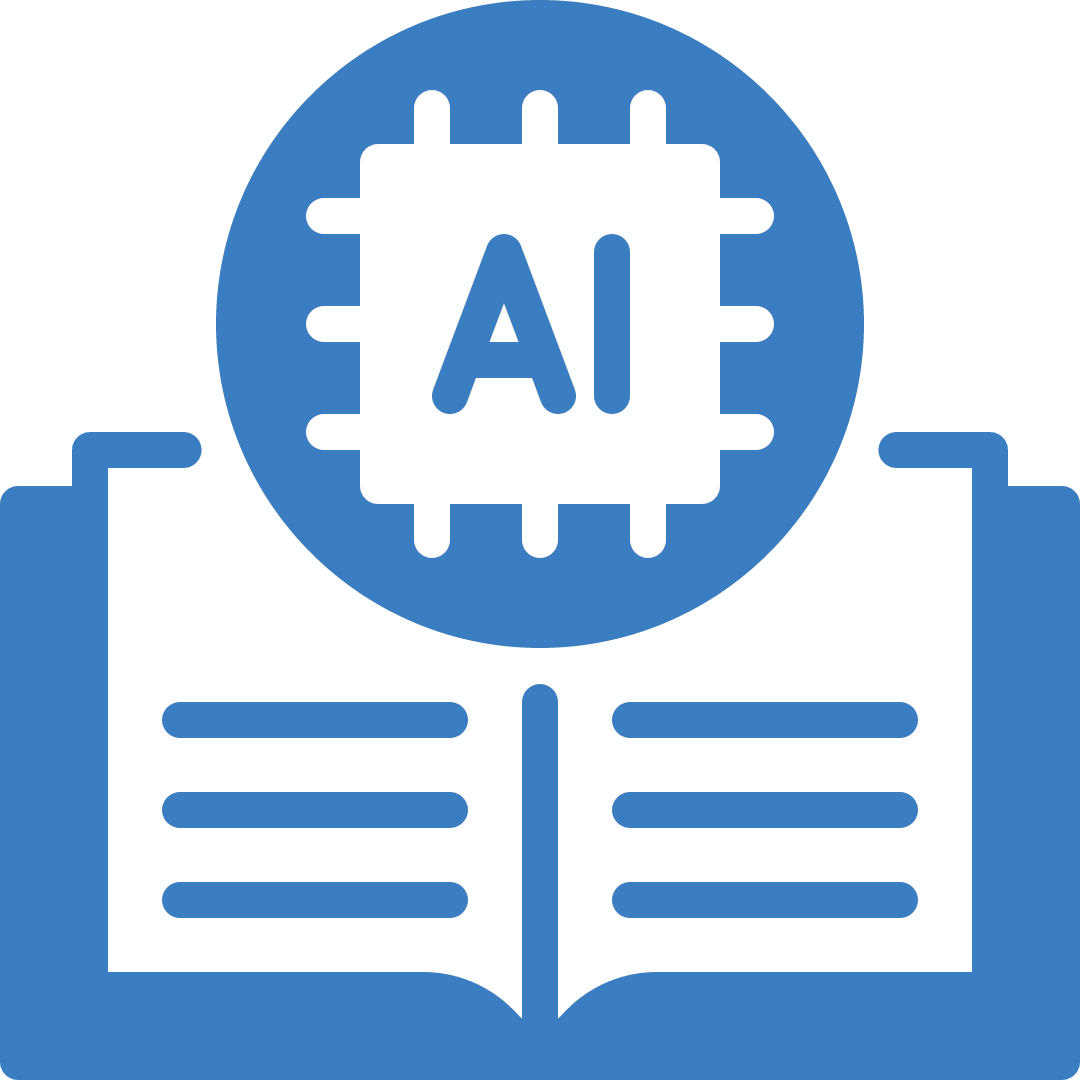
As AI technologies continue to mature, the collaboration between artificial intelligence and software quality assurance promises a future where software is not just developed but is developed with quality at its core.
Looking ahead, the future of SQA is intertwined with AI. Emerging trends, including natural language processing and AI-driven analytics, promise further advancements. The symbiotic relationship between AI and SQA is poised to redefine how we approach software quality.
The infusion of AI into SQA processes is not a singular act but a multi-faceted transformation. It reshapes the very fabric of how testing is approached, moving from rigid structures to adaptive methodologies. The inherent learning capabilities of AI mean that testing processes evolve, learning from each iteration and becoming more attuned to the nuances of the software under scrutiny.
Human Intervention in SQA
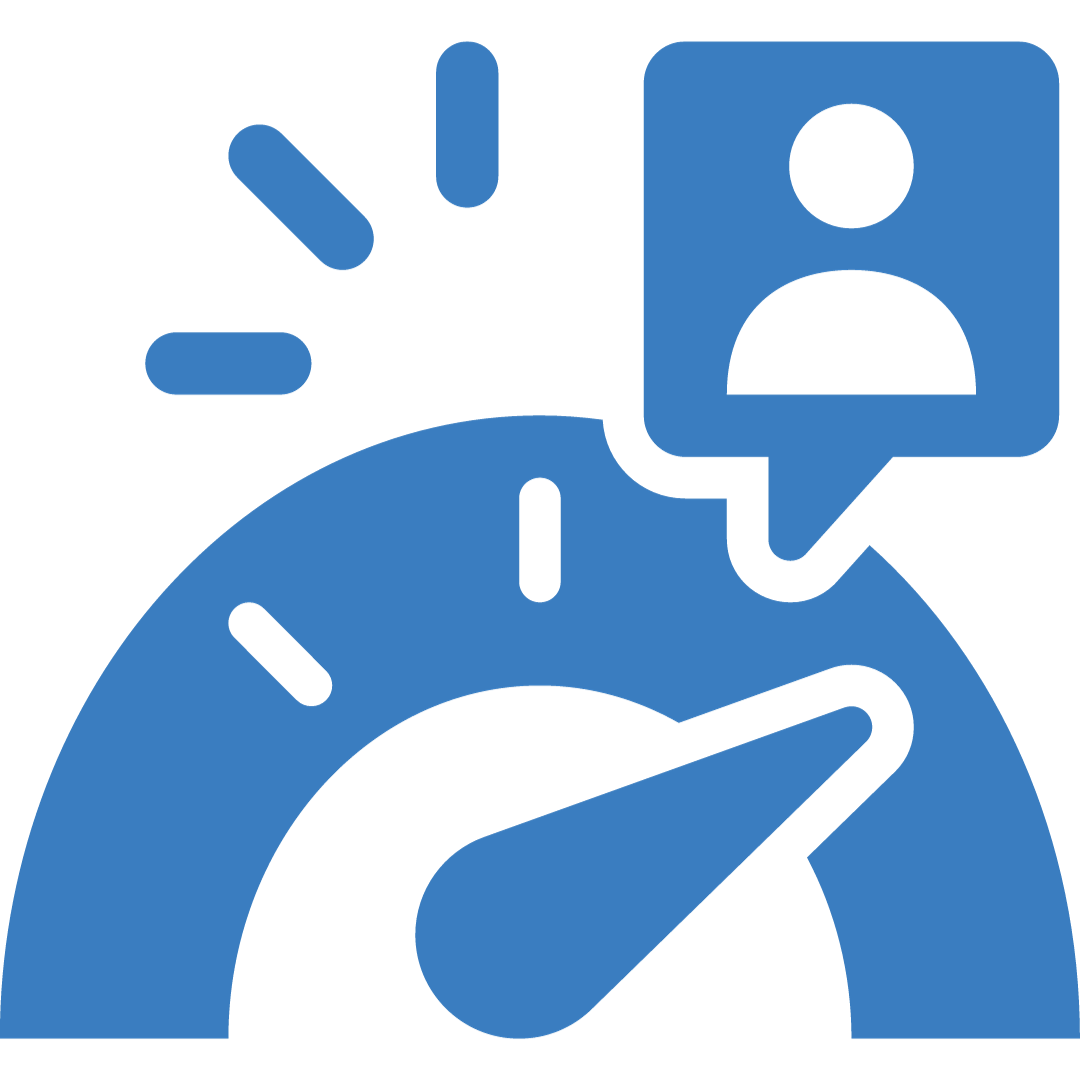
One cannot overlook the profound impact on the human element in SQA. As AI takes on the repetitive and time-consuming aspects of testing, human testers are liberated to focus on strategic and creative elements. This shift in roles allows human testers to apply their cognitive abilities to areas that demand intuition, critical thinking, and a deep understanding of user experiences, amplifying the overall effectiveness of the quality assurance process.
Looking ahead, the trajectory of AI in SQA is one of ongoing exploration. Natural language processing, cognitive computing, and the evolution of AI models present a future where the interaction between human testers and AI becomes even more intuitive. The roadmap ahead is not just about refining processes but about fostering a symbiotic relationship where AI augments human capabilities to create software that not only meets but exceeds the expectations of users.
In conclusion, the narrative of enhancing Software Quality Assurance through AI integration is not a story with a fixed endpoint but an ongoing saga of innovation. It's a testament to the resilience of the software development community in embracing new paradigms. The journey continues, and the marriage of AI and SQA promises a future where the quality of software is not just assured but is an ever-evolving benchmark of excellence. To know more, get in touch with our QA experts!
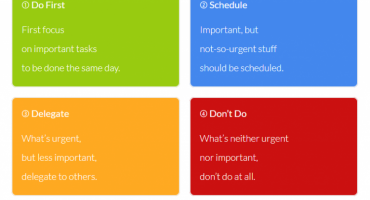
Be productive using tools and techniques.
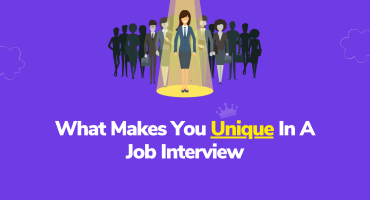
What Makes You Unique In A Job Interview
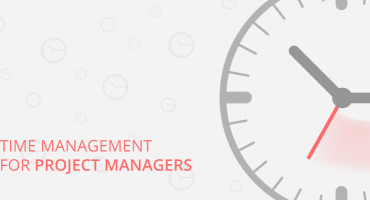