How Artificial Intelligence Is Impacting Quality Assurance Roles: Part 1
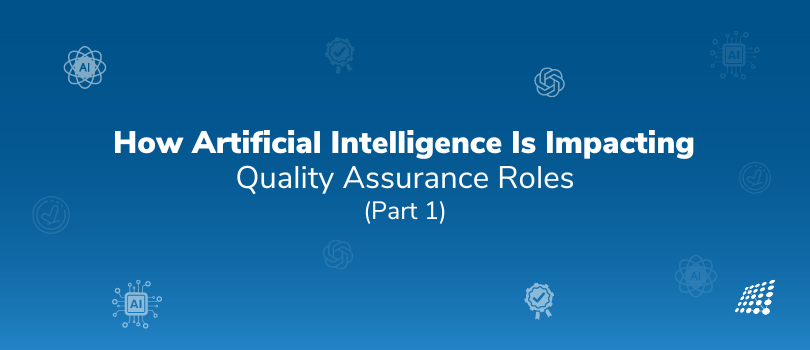
At the core of technological reliability, Quality Assurance (QA) has long been an imperative in releasing error-free software. With the rise of Artificial Intelligence (AI) in QA, we're witnessing a dramatic transformation that boosts both the precision and the speed of these processes. "How does AI redefine the roles within QA?" you might wonder.
In our series "How Artificial Intelligence Is Impacting Quality Assurance Roles: Part 1," we take a closer look at AI in quality assurance, how AI's integration is reshaping test automation, sharpening predictive analysis, and redefining exploratory testing. Discover with us the ways AI is not only tweaking existing QA processes but also carving out new roles within the field.
The Influence of AI on Test Automation Roles
Here is one of the examples of AI automation and its powerful influence:
Let me tell you about a project that really put our skills to the test. We faced a tough challenge: ensure a complex app, with both web and mobile versions, was thoroughly tested within two-week release cycles. We had to quickly and thoroughly test new features and ensure existing functionalities were not affected while maintaining consistency between the web and mobile versions. Our manual testing, especially the 80 hours spent on regression tests, just wasn't sustainable given the pace.
The turning point was when we introduced test automation using Selenium and Java. Selenium's versatility meant we could reliably test across different environments and keep the web and mobile experiences consistent.
This move drastically cut down our regression testing time from 80 hours to just a few. It freed up the team to focus on more challenging tasks and kept us on track for each release, without compromising the app's quality.
Embracing test automation showed us its true potential for complex projects, boosting our efficiency and ensuring effective testing without fail.
- Paresh Bagi, Assistant Manager (Technical)
How Machine Learning Creates Tests
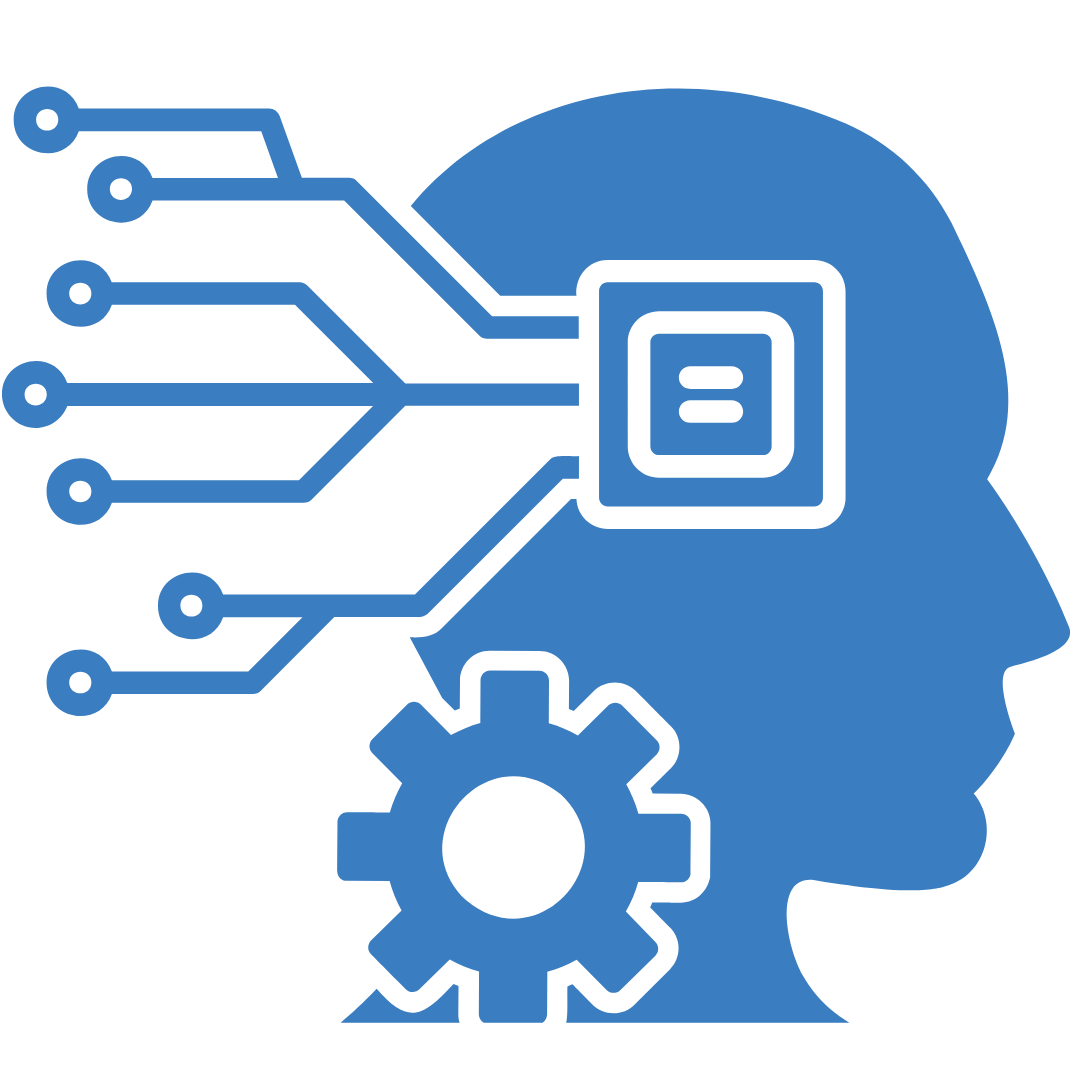
Machine Learning (ML) algorithms have reinvented the way test cases are created. Now, it’s about analyzing software behavior and utilizing insights from past tests to develop robust and adaptive test scenarios. This adaptability is crucial, as it ensures that test suites remain effective even as applications evolve, making machine learning for testing an essential tool in the ongoing process of test suite enhancement.
Boosting Test Automation with AI
Embedding AI within test automation refines efficiency and marks a leap in testing capabilities, with improvements seen in the scope of testing and the fineness of the results.
- AI enables the detection of complex connections within the product's functions, ensuring a more thorough testing approach.
- The capacity for real-time adjustment to software changes through AI-driven scripts further enhances the maintenance process.
Leading AI Test Automation Tools
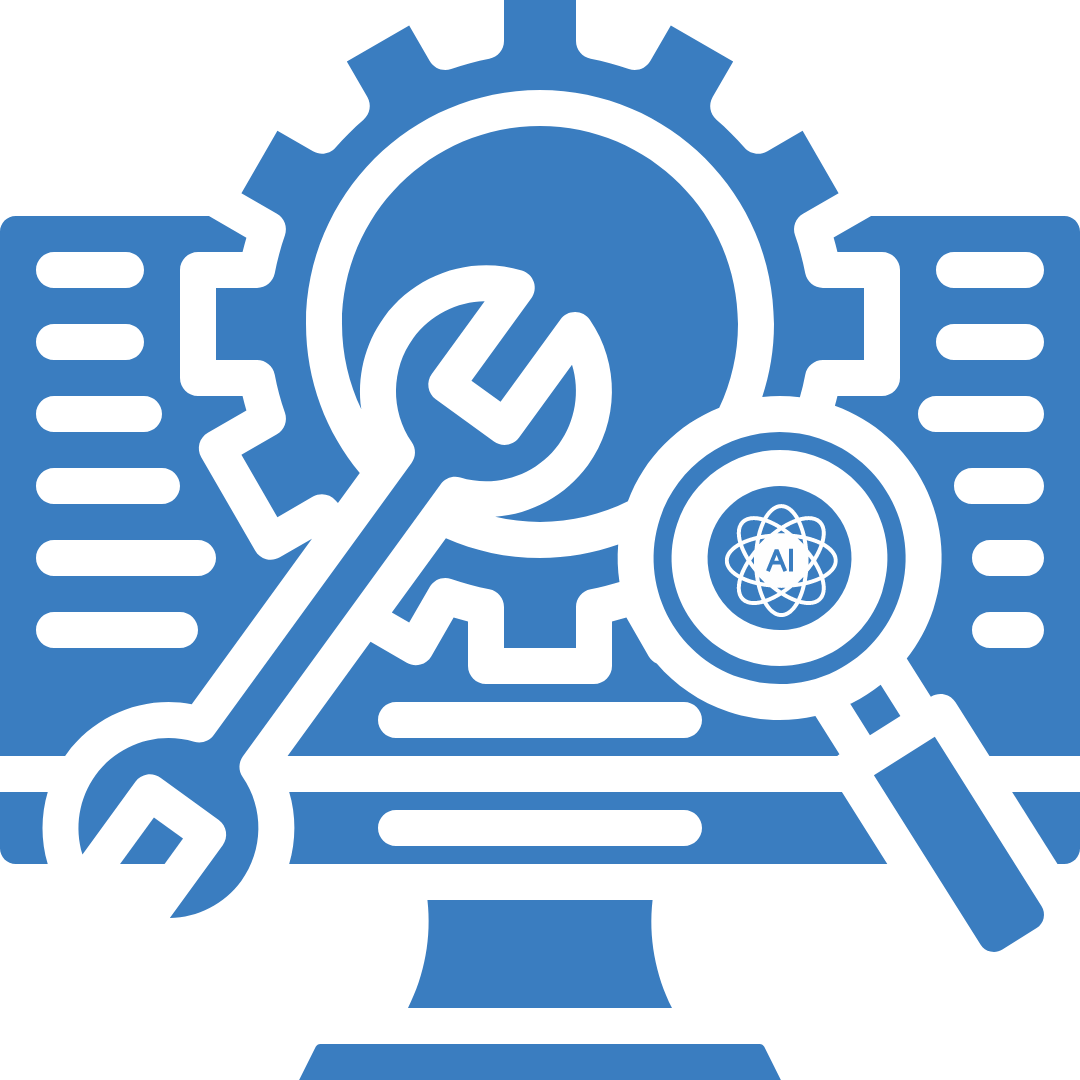
The marketplace offers a variety of artificial intelligent test automation tools, including:
- Applitools: Employs AI to conduct precise visual tests and spot UI modifications.
- Testim: Utilizes ML for the generation and upkeep of test scripts.
Other AI Test Automation Tools Worth Trying:
- Mabl: Supports self-governing testing with the power of AI.
- Functionize: Deploys ML for the autonomous repair of test scripts.
- TestCraft: Integrates AI with codeless automation for improved efficiency.
Predictive Analysis Elevating Software Quality Assurance (SQA)
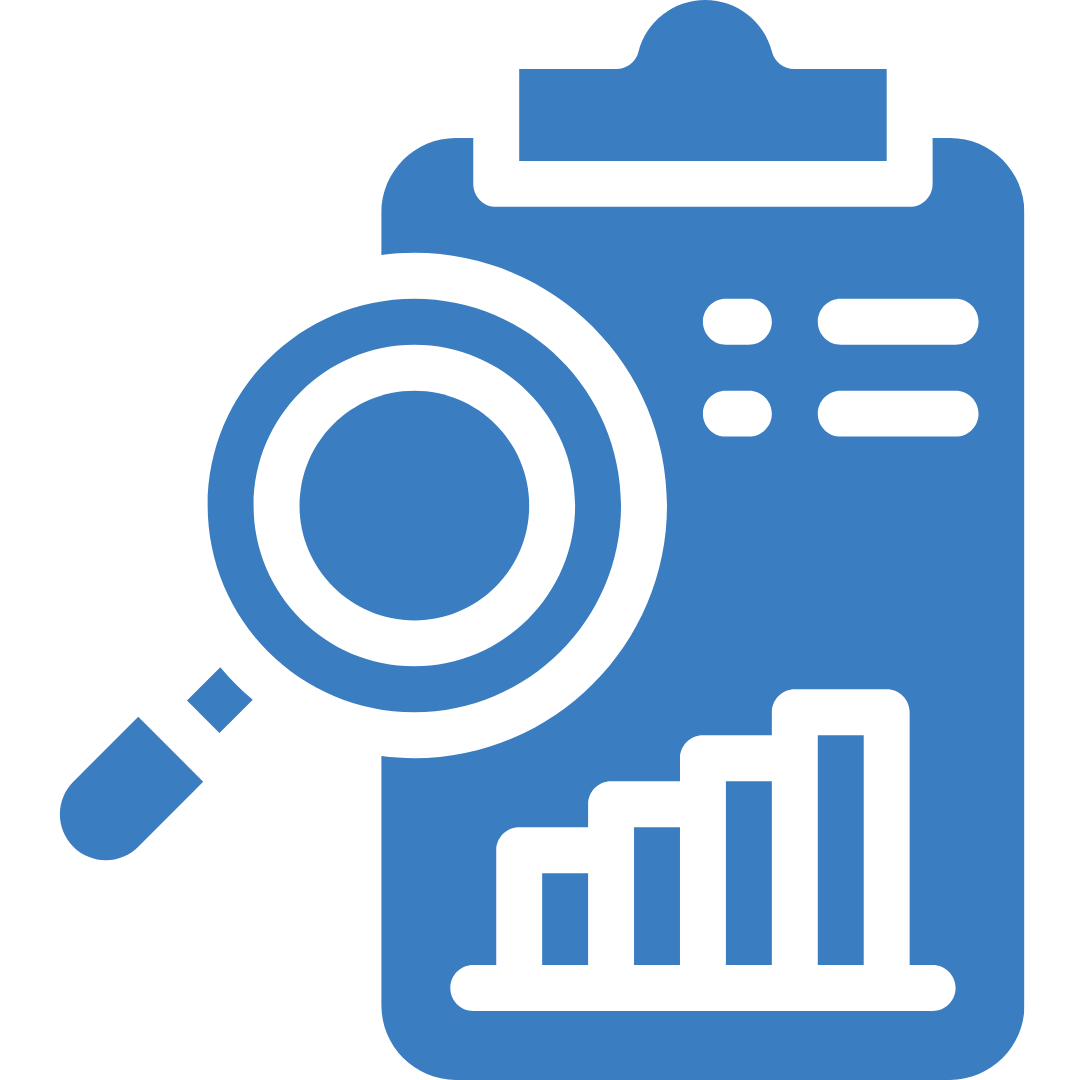
Predictive analysis in Software Quality Assurance (SQA) employs the power of data to forecast potential issues, enabling teams to address them preemptively. This analytical approach accompanied by predictive analysis tools strengthens the SQA process, empowering teams to ensure product excellence well before software reaches the end user.
The Integration of AI in SQA
The fusion of AI with SQA has brought about a considerable reduction in human error, making the QA cycle more efficient and less time-consuming. AI boosts SQA in various ways:
Advantages of AI-Powered SQA
- Accuracy: AI's ability to process complex data leads to more precise outcomes.
- Speed: By working quickly, AI cuts down the time from software development to delivery.
- Adaptability: AI continually improves its functions by learning from data, becoming more efficient over time.
Challenges in AI Application
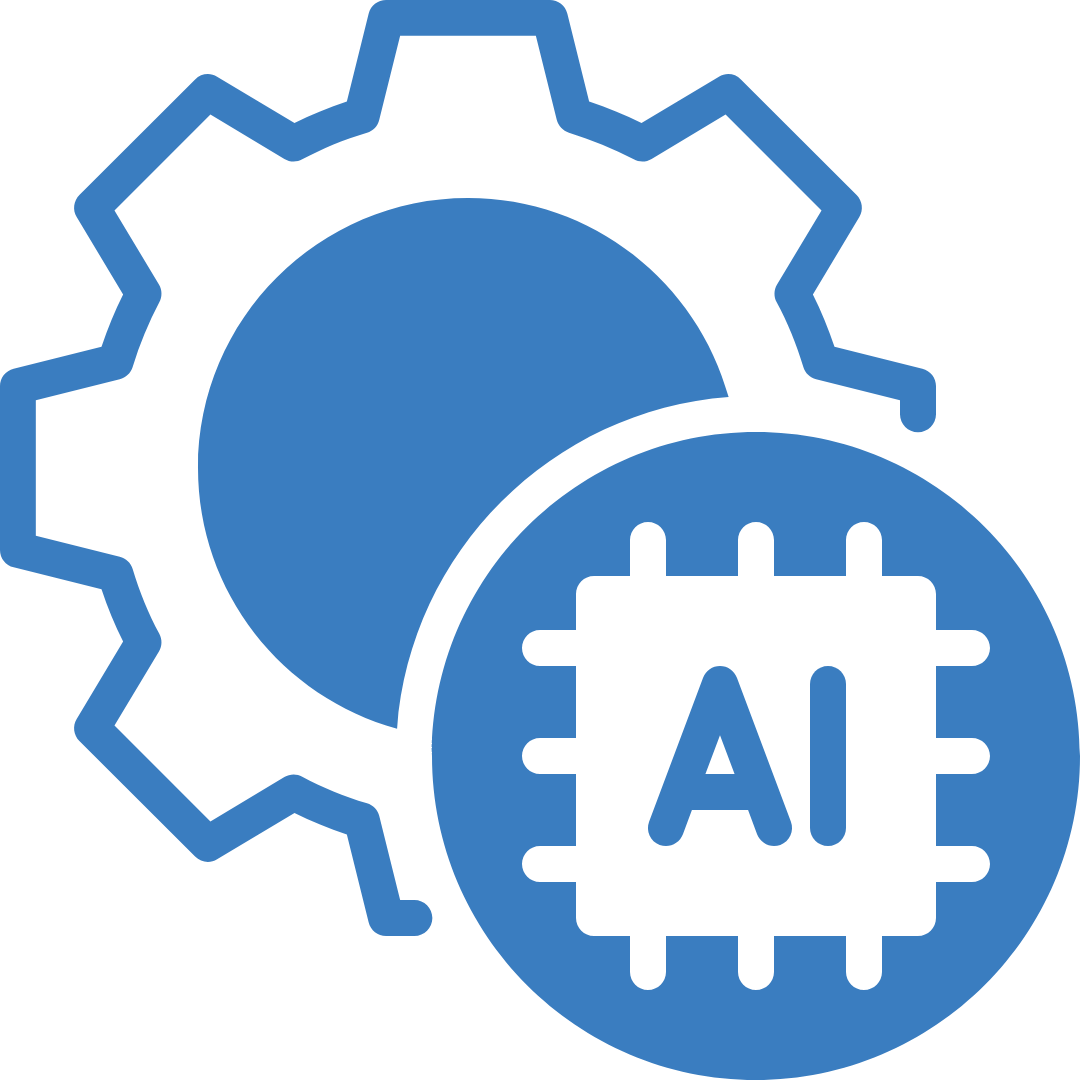
However, there are also challenges to AI adoption when integrating AI into SQA operations:
- Initial Investment: The cost of deploying AI solutions may be high at the outset, though this is expected to reduce as the technology evolves.
- Data Reliance: The effectiveness of AI's predictions depends on the quality of the input data, which requires careful management.
- Trust: Given that AI technology is still developing, there is a need for vigilant oversight from skilled professionals to identify and correct issues that AI might overlook.
In industries ranging from e-commerce to healthcare, AI’s role in predictive analysis has become indispensable, bolstering the SQA mission to deliver flawless software functionality reliably to consumers.
AI's Advancements in Exploratory Testing
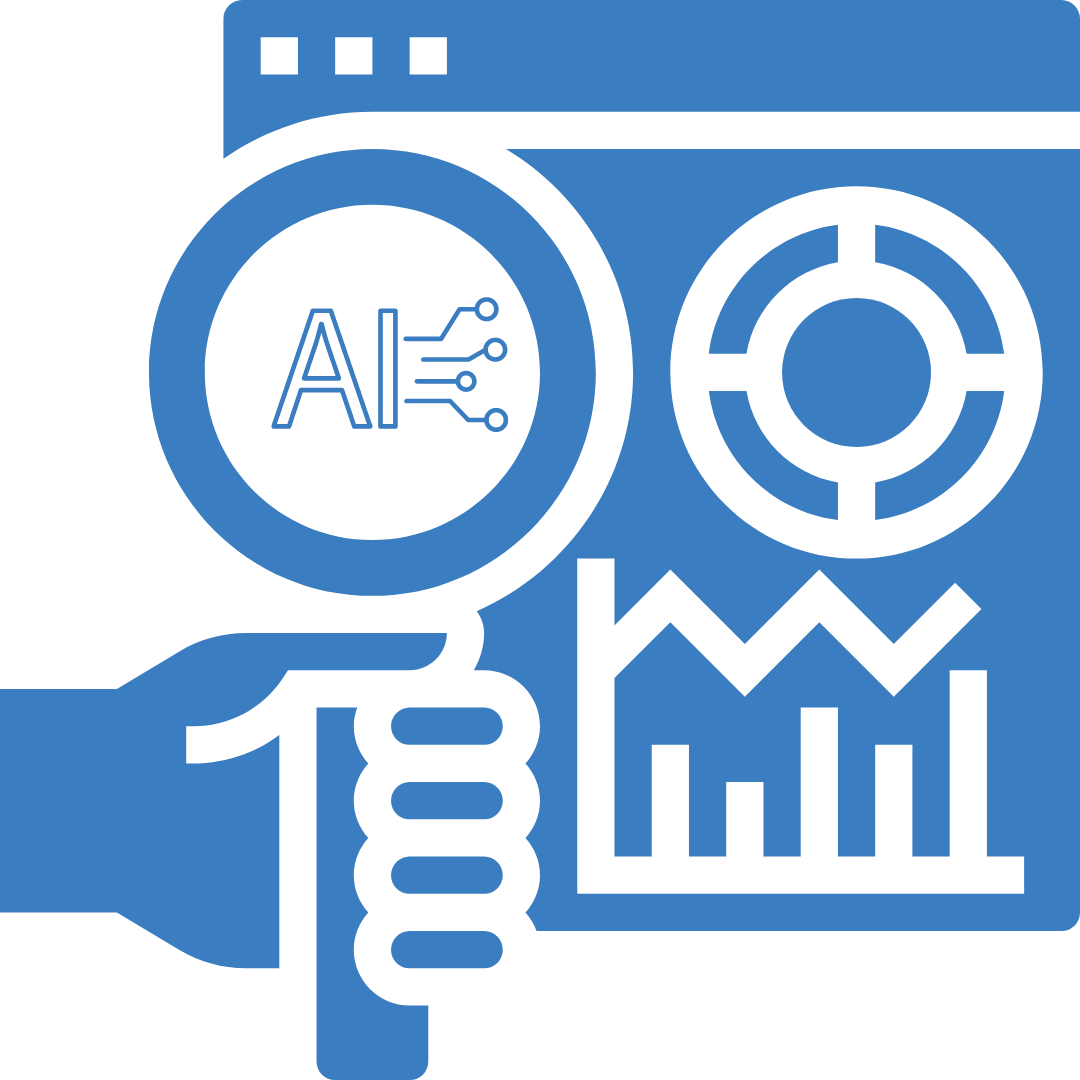
AI technology is bringing a new level of sophistication to exploratory testing methods, a traditionally agile and hands-on process that relies heavily on a tester's experience and intuition to uncover flaws and assess user interaction.
The Imperative for AI in Exploratory Testing
With software becoming more intricate than ever, AI's role in exploratory testing has grown from a luxury to a necessity, offering precision and streamlining workflows where they're most needed.
AI-Powered Test Case Generation
- Automated Insight: AI scours through lines of code and historical data, employing ML algorithms to discern and prioritize the most critical testing scenarios.
- Focused Testing: This enables testers to target the most vital areas of an application, ensuring thorough examination where it matters most.
Data-Enriched Testing Insights
- Pattern Recognition: AI taps into expansive datasets accumulated during testing phases, unearthing trends and potential issues that might slip past manual review.
- Data-Driven Decisions: This results in a more comprehensive and informed testing strategy, rooted in robust data analysis.
Streamlining Test Execution
- Efficiency Boost: By automating the more routine aspects of testing, AI allows human testers to allocate more time to the complex, creative facets of exploratory testing.
- Real-Time Detection: AI's continuous monitoring also enables the immediate identification of new or emerging issues throughout the testing process.
Elevating Test Coverage
- Gap Identification: AI's thorough evaluation of test coverage ensures that no part of the application is left unchecked, guaranteeing a more extensive and fail-safe validation.
How AI is Affecting User Experience?
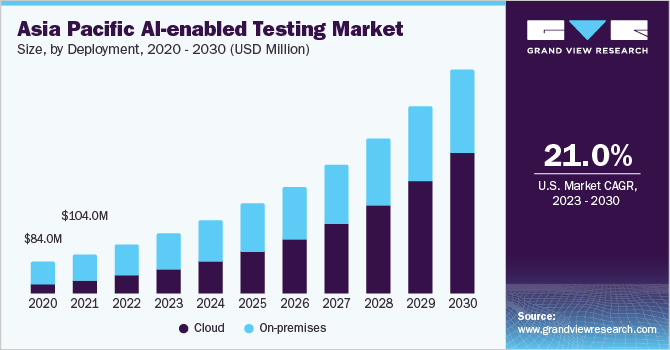
Various factors influence user experience. Through the power of AI, we can now predict software defects by meticulously scanning past data, which helps us channel our testing efforts effectively toward the most susceptible areas. An illustrious report by Grand View Research shines a light on the burgeoning market for AI-enhanced testing tools. As of 2022, this market boasted a size of USD 414.7 million. Looking ahead, we can expect an impressive growth trajectory with a compound annual growth rate of 18.4% projected from 2023 to 2030.
AI doesn't stop there – it revolutionizes the way we report bugs. With intelligent systems, we gather critical bug data automatically, while AI prioritizes these bugs, sorting them by their severity and potential impact on the system. Furthermore, AI's vigilant real-time monitoring spots inconsistencies and emerging patterns, paving the way for preemptive measures against potential issues. To know how AI in QA is making an impact more extensively, get in touch with our QA experts now!
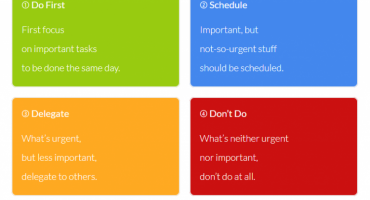
Be productive using tools and techniques.
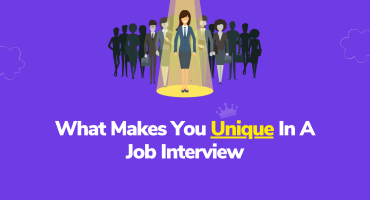
What Makes You Unique In A Job Interview
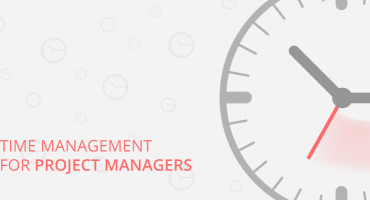